Healthcare analytics is revolutionizing the medical field, offering unprecedented insights into patient care, operational efficiency, and disease management. By leveraging data, healthcare providers can make informed decisions that enhance patient outcomes, reduce costs, and streamline operations. This article explores the key aspects of healthcare analytics, including its importance, types, applications, challenges, and future trends.
The Importance of Healthcare Analytics
Healthcare statistics is crucial for several reasons, impacting both clinical and administrative aspects of healthcare.
Improving Patient Outcomes: Analytics enables healthcare providers to identify patterns and predict outcomes, allowing for proactive interventions. For example, predictive analytics can foresee patient deterioration, enabling timely treatment that can save lives.
Cost Reduction: By analyzing operational data, healthcare organizations can identify inefficiencies and implement cost-saving measures. This includes optimizing resource allocation, reducing unnecessary tests and procedures, and preventing hospital readmissions.
Enhanced Decision-Making: Data-driven decision-making is more accurate and reliable. Healthcare analytics provides clinicians and administrators with real-time data and insights, leading to better-informed decisions regarding patient care and resource management.
Personalized Medicine: Analytics supports personalized medicine by analyzing genetic, clinical, and lifestyle data to tailor treatments to individual patients. This approach improves the effectiveness of treatments and reduces adverse effects.
Regulatory Compliance: Healthcare statistics help organizations comply with regulations by providing comprehensive data tracking and reporting capabilities. This ensures adherence to standards such as HIPAA and GDPR.
Types of Healthcare Analytics
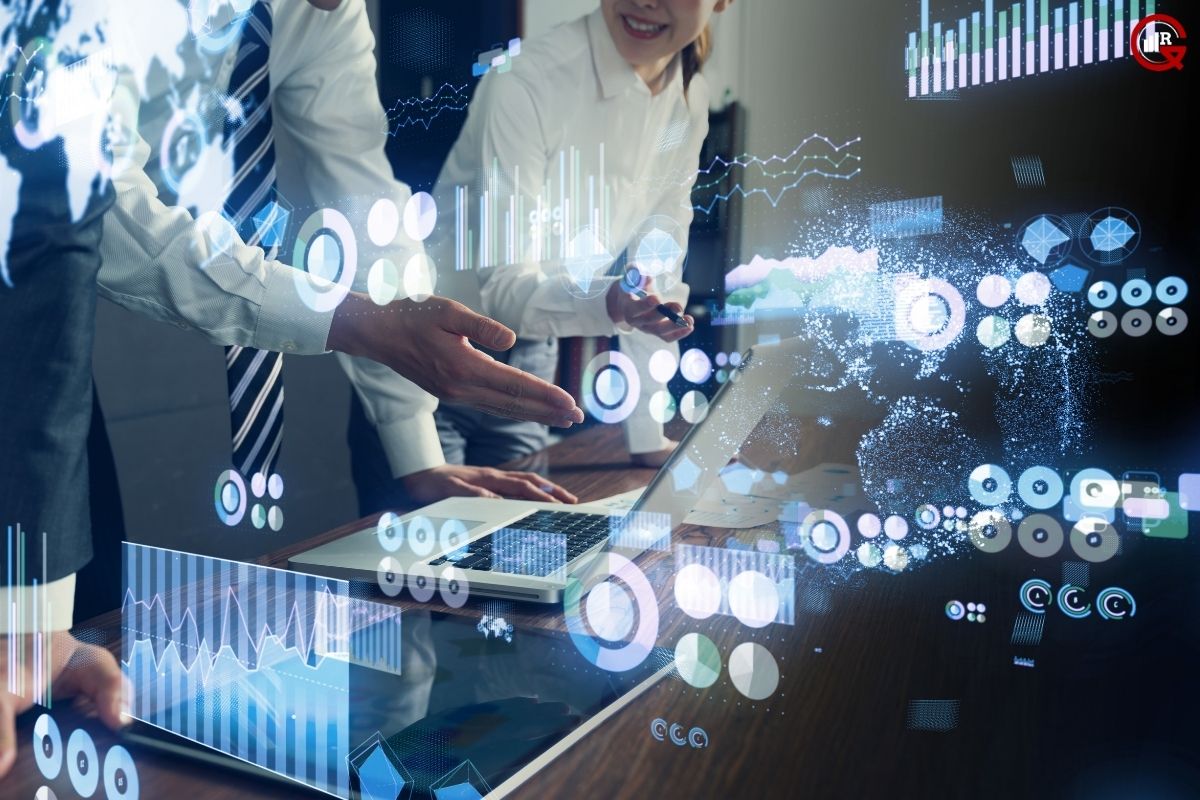
Healthcare statistics can be categorized into several types, each serving distinct purposes and providing unique insights.
Descriptive Analytics: This type involves analyzing historical data to understand what has happened in the past. It includes the use of dashboards and reports to monitor key performance indicators (KPIs) and other metrics. Descriptive analytics helps healthcare providers understand trends and patterns in patient care and operational performance.
Diagnostic Analytics: Diagnostic analytics delves deeper into data to determine the causes of past events. It uses techniques such as data mining, correlation analysis, and root cause analysis to uncover the reasons behind specific outcomes. This helps healthcare organizations understand the factors contributing to patient outcomes and operational inefficiencies.
Predictive Analytics: Predictive analytics uses statistical models and machine learning algorithms to forecast future events based on historical data. In healthcare, it can predict disease outbreaks, patient readmissions, and treatment responses. This proactive approach enables healthcare providers to take preventive measures and improve patient care.
Prescriptive Analytics: This advanced type of analytics suggests actions based on predictive insights. By combining data analysis with decision science, prescriptive analytics recommends the best course of action to achieve desired outcomes. It can optimize treatment plans, resource allocation, and operational workflows.
Cognitive Analytics: Cognitive analytics leverages artificial intelligence (AI) and natural language processing (NLP) to mimic human thought processes. It can analyze unstructured data such as clinical notes and research papers, providing deeper insights and supporting complex decision-making in healthcare.
Applications of Healthcare Analytics
Healthcare statistics has a wide range of applications that significantly impact various aspects of the healthcare industry.
Clinical Decision Support: Analytics can assist clinicians in making evidence-based decisions by providing insights from large volumes of clinical data. Decision support systems can offer recommendations for diagnosis and treatment, helping to improve patient outcomes and reduce medical errors.
Population Health Management: By analyzing demographic and clinical data, healthcare organizations can identify high-risk populations and develop targeted interventions. Population health analytics helps in managing chronic diseases, reducing health disparities, and improving community health.
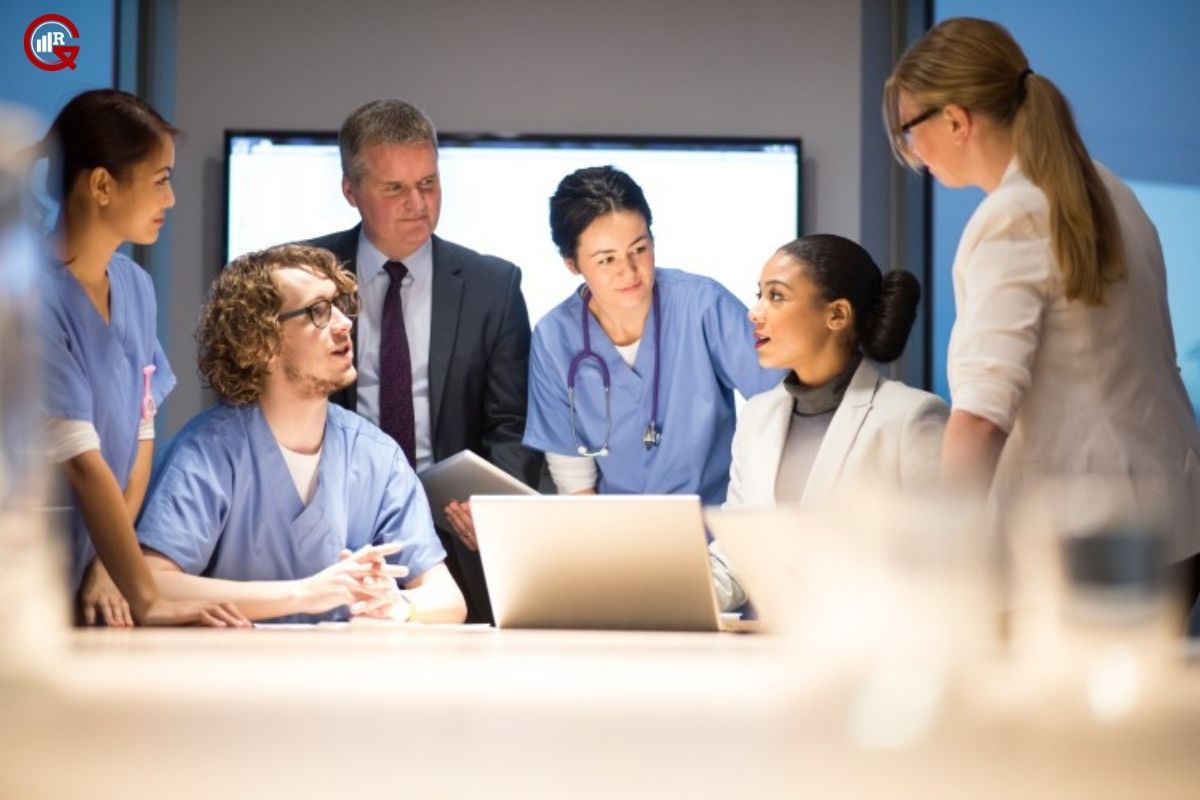
Operational Efficiency: Healthcare analytics can optimize hospital operations by improving scheduling, resource utilization, and supply chain management. For example, predictive analytics can forecast patient admission rates, enabling better staffing and resource allocation.
Fraud Detection and Prevention: Analytics can identify patterns indicative of fraudulent activities, such as billing inconsistencies and unusual claim patterns. This helps healthcare organizations prevent fraud, reduce financial losses, and ensure compliance with regulatory standards.
Research and Development: In medical research, analytics plays a critical role in drug discovery, clinical trials, and epidemiological studies. By analyzing vast amounts of data, researchers can identify potential treatments, monitor disease outbreaks, and accelerate the development of new therapies.
Challenges in Implementing Healthcare Statistics
Despite its benefits, the implementation of healthcare analytics faces several challenges that need to be addressed for its successful adoption.
Data Quality and Integration: Healthcare data often comes from diverse sources, including electronic health records (EHRs), laboratory systems, and wearable devices. Ensuring data quality and integrating these disparate data sources is a significant challenge.
Privacy and Security: Handling sensitive patient data requires stringent privacy and security measures. Ensuring compliance with regulations such as HIPAA while protecting against data breaches is critical for maintaining patient trust and avoiding legal repercussions.
Interoperability: Different healthcare systems and platforms often use incompatible data formats and standards, making data exchange difficult. Achieving interoperability is essential for seamless data sharing and comprehensive analytics.
Skill Gaps: There is a shortage of skilled professionals who can effectively analyze healthcare data and interpret the results. Bridging this skill gap through education and training is necessary for the successful implementation of analytics in healthcare.
Cost and Infrastructure: Implementing advanced analytics solutions requires significant investment in technology and infrastructure. Smaller healthcare organizations may struggle with the financial burden, making it essential to find cost-effective solutions and partnerships.
Future Trends in Healthcare Analytics
The future of healthcare analytics is promising, with several emerging trends set to transform the industry further.
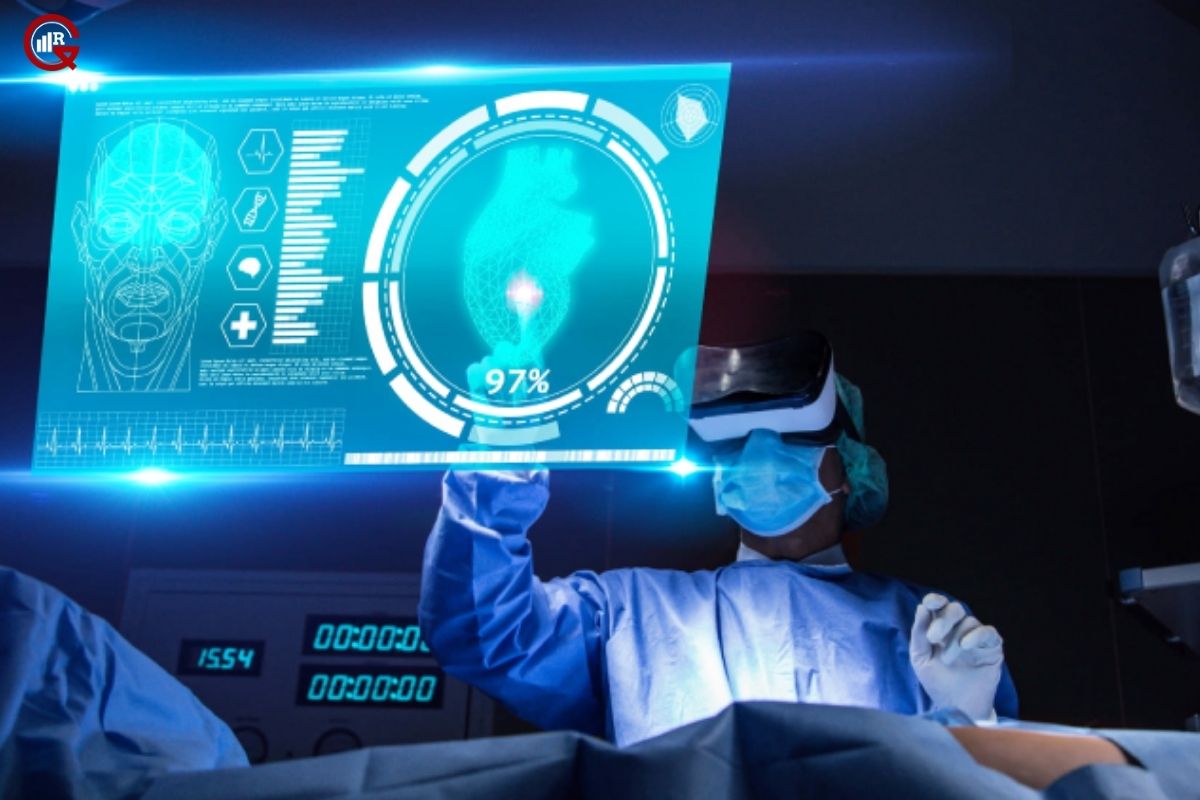
AI and Machine Learning: The integration of AI and machine learning in healthcare statistics will enhance predictive and prescriptive capabilities. These technologies can process large datasets quickly, uncover hidden patterns, and provide more accurate forecasts and recommendations.
Real-Time Analytics: The demand for real-time data analysis is growing. Real-time analytics can provide immediate insights into patient care and operational performance, enabling faster decision-making and more responsive healthcare delivery.
Personalized and Precision Medicine: Advances in genomics and molecular biology are driving the growth of personalized and precision medicine. Analytics will play a crucial role in tailoring treatments to individual patients based on their genetic makeup and other factors.
Patient Engagement and Remote Monitoring: Wearable devices and mobile health apps are generating vast amounts of data that can be used for remote patient monitoring and engagement. Analytics can help interpret this data, providing insights that empower patients to take an active role in managing their health.
Blockchain for Data Security: Blockchain technology offers a promising solution for enhancing data security and interoperability in healthcare. By providing a decentralized and tamper-proof ledger, blockchain can ensure secure and transparent data sharing across the healthcare ecosystem.
In conclusion, healthcare analytics is transforming the medical field by providing valuable insights that improve patient care, operational efficiency, and overall healthcare outcomes. While challenges remain, ongoing advancements in technology and data science are paving the way for a more data-driven and efficient healthcare system. By embracing these innovations, healthcare providers can deliver better care, reduce costs, and ultimately improve the health and well-being of populations.